December 02, 2020
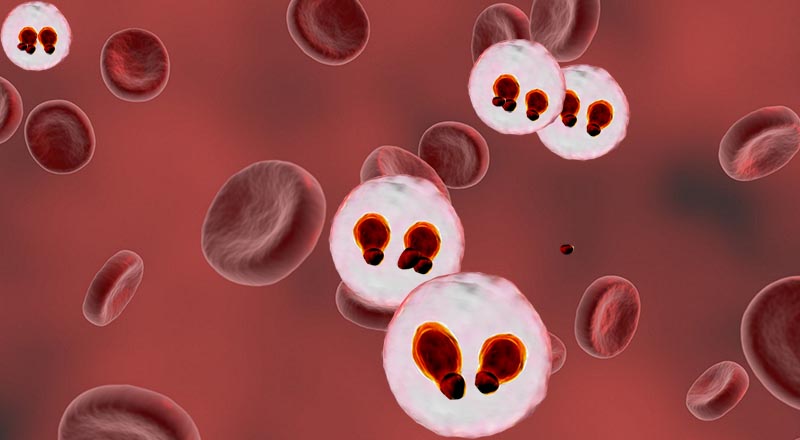
This illustration of red blood cells shows cells infected with the malaria parasite.
Mizzou Engineers are part of a global team developing a smarter way to combat malaria—a leading cause of death in the world. Now, they’ve outlined a two-step approach that will use deep learning to more accurately and efficiently diagnose the disease.
“According to the World Health Organization, a child dies every two minutes, because of malaria,” said Yasmin Kassim, who earned her PhD in Electrical and Computer Engineering in May 2020. “Our automated algorithms assist microscopists in the field, and in primary care in resource-poor settings to have a better way to read blood smears and provide fast and reliable results. Researchers and doctors in major hospitals and research institutes could benefit from this as well, although they are not the targeted end users.”
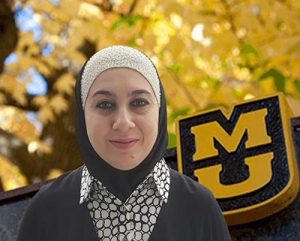
Kassim
Kassim is working with EECS Professor Kannappan Palaniappan, and researchers from the National Institutes of Health and Oxford University.
The team published their most recent findings in the Institute for Electrical and Electronics Engineers Journal of Biomedical and Health Informatics. The paper is titled “Clustering-Based Dual Deep Learning Architecture for Detecting Red Blood Cells in Malaria Diagnostic Smears.”
While a PhD student under Palaniappan’s supervision, Kassim helped design the dual deep learning framework to accurately count cells in thin blood smears through advanced imaging. The first super-pixel architecture identifies red blood cell clusters that may be densely grouped or touching, while the second stage extracts individual cells and filters out white blood cells.
RBCNet allows each architecture backbone of the deep learning pipeline to specialize and cooperatively segment hundreds of cells, even up to a thousand objects in each image very accurately, which is a challenging requirement, Palaniappan said.
“Deep learning doesn’t always work right out of the box,” he said. “Our previous active contour cell segmentation approach does very well. In order to improve upon existing methods you have to have insight not to over train an algorithm. Incorporating a second stage is a chance to recover from errors, so you’re not forcing one algorithm to do everything.”
A Smarter Way to Screen
Currently, diagnosing malaria involves manually counting parasites in infected red blood cells in blood smear samples. The process is critical to determining the best treatment strategies for a patient.
But it has significant limitations. Because it requires manual labor, millions of blood smears wait too long to be diagnosed. The process is time consuming and costly. And because it’s manual, it’s not standardized and is subject to human error.
Palaniappan has been working with others to develop an automated approach since 2013. Primarily, he’s been working with Stefan Jaeger, a researcher at the Lister Hill National Center for Biomedical Communications at the U.S. National Library of Medicine, part of the National Institutes of Health and Richard Maude an Associate Professor in the Nuffield Department of Medicine at Oxford University.
The goal has been to train deep learning systems to recognize the shape and appearance of single cell Plasmodium parasites and let machine learning count them and detect which red blood cells have been infected.
The team has since developed a mobile software application to do this. Malaria Screener runs on a common smartphone that is attached to a microscope, and uses the phone’s camera to capture blood smear images.
The smartphone app is being tested for malaria diagnosis in a clinical field study being carried out by the Foundation for Innovative New Diagnostics (FIND), Jaeger said. Additionally, researchers are testing the app in several other countries.
The app is expected to provide a more reliable way to diagnose malaria and reduce diagnostic costs.
Kassim got involved in the work when she was selected for the NIH Medical Informatics Training program summer internship with NLM during the summer of 2018—four years after joining the Electrical and Computer Engineering PhD program at Mizzou.
During her involvement, the team tested the dual deep learning architecture using human malaria smears acquired in Bangladesh. Cell detection accuracy was higher than 97%.
Now, she’s looking forward to seeing the work have an impact on human lives.
“There are countries that have millions of smears that need to be read by microscopists,” Kassim said. “We want to assist them and build automated algorithms that provide fast, inexpensive results, with accurate readings on infection rates for those slides. Automated malaria diagnosis can improve and speed up treatment for this deadly disease.”