March 25, 2019
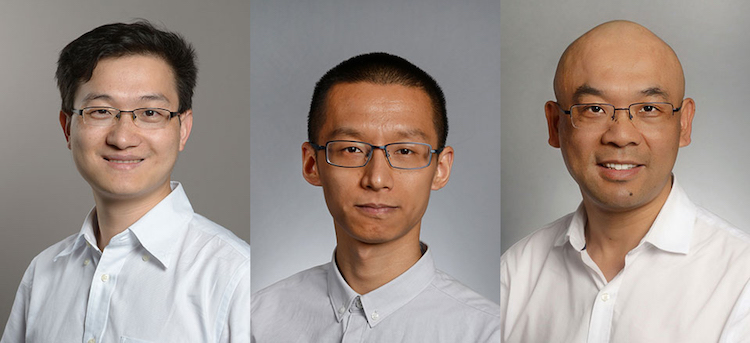
Jian Lin, Yuan Dong and Jianlin Cheng led the team that recently published “Bandgap prediction by deep learning in configurationally hybridized graphene and boron nitride,” in the high-impact journal npj Computational Materials.
Graphene is a single layer of carbon atoms that has a wide array of potential uses, particularly as a candidate material for use in electronic devices, such as LED screens, touch panels, smart phones and solar cells.
Graphene’s electrical and optical properties can be significantly altered for better usage. Discovering how these atoms tune to create these properties is one of the most pressing questions in materials science. At Mizzou Engineering, a research team is one of the first to significantly expedite the process by applying the same principles — known as deep learning — that allow computers to learn by example. It’s the technology used in language recognition, housekeeping robots, autonomous vehicles, news aggregators and more, to the field of materials science.
Jian Lin, assistant professor of Mechanical and Aerospace Engineering; Jianlin Cheng, William and Nancy Thompson Professor of Electrical Engineering and Computer Science; research assistant professor Yuan Dong; and students Chuhan Wu, Chi Zhang and Yingda Liu formed the interdepartmental team that recently published “Bandgap prediction by deep learning in configurationally hybridized graphene and boron nitride,” in the high-impact journal npj Computational Materials.
“If you put atoms in certain configurations, when you apply certain stipulations to the electrical field, the material will behave differently. Structures determine the properties. How can you predict these properties without doing experiments? That’s where computational principles come in,” Lin, who conceived the project, explained.
Experiments are time-consuming and expensive, and merely scratch the surface of the billions of structural possibilities of graphene. By utilizing deep learning models, researchers bypass this issue to predict which configurations of boron and nitrogen atoms in graphene will produce the necessary properties at more than 95 percent accuracy.
The ability of deep learning was critical to this interdisciplinary research. Lin’s lab designed the material descriptor for the deep learning models so that a material problem can be successfully converted to a computer problem, and they also provided a couple of thousand known combinations of graphene structures and their properties to serve as datasets for the high-performance computing array.
Cheng’s lab then input this data into the models, allowing the high-performance computer to learn from these examples and extrapolate patterns, which then can be used to predict the properties of the billions of other possible structures without having to test each one individually.
“If you have a good experimental materials system, you can train a computer to do what it would take many years to otherwise do,” Dong said. “This is a good starting point.”
Deep learning techniques have been around for several years. However, they have not often — if ever — been utilized in the realm of materials science. The hope is that the example produced at Mizzou Engineering can serve as the start of a wealth of research on utilizing deep learning in relation to other types of materials, greatly advancing research at a rapid rate.
“You train an intelligent computer system and give it any design, and it can predict the properties. This trend is emerging in the material science field,” Cheng said. “It’s a great example of applying artificial intelligence to changing the material design paradigm in this field. It’s great interdisciplinary research.”